Master Vector Database with Python for AI & LLM Use Cases
- Description
- Curriculum
- FAQ
- Reviews
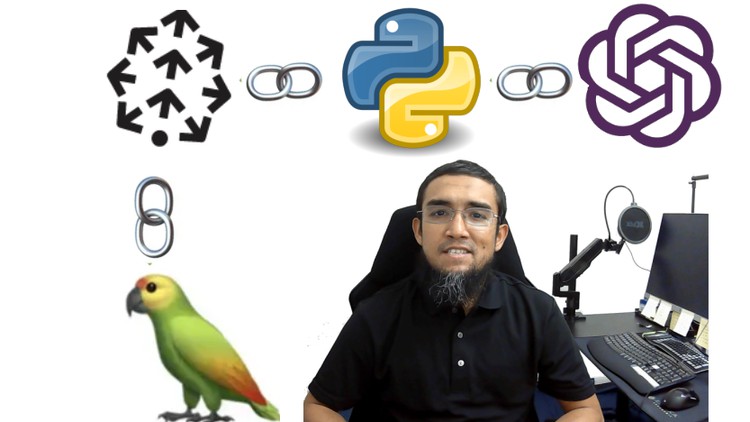
In this comprehensive course on Vector Databases, you will delve into the exciting world of cutting-edge technologies that are transforming the field of artificial intelligence (AI), particularly in generative AI. With a focus on Future-Proofing Generative AI, this course will equip you with the knowledge and skills to harness the power of Vector Databases for advanced applications, including Language Model Models (LLM), Generative Pretrained Transformers (GPT) like ChatGPT, and Artificial General Intelligence (AGI) development.
Starting from the foundations, you will learn the fundamentals of Vector Databases and their role in revolutionizing AI workflows. Through practical examples and hands-on coding exercises, you will explore techniques such as vector data indexing, storage, retrieval, and conditionality reduction. You will also gain proficiency in integrating Pinecone Vector Data Base with other tools like LangChain, OpenAI API using Python to implement real-world use cases and unleash the full potential of Vector Databases.
Throughout the course, we will uncover the limitless possibilities of Vector Databases in generative AI. You will discover how these databases enable content generation, recommendation systems, language translation, and more. Additionally, we will discuss performance optimization, scalability considerations, and best practices for efficient implementation.
Led by an expert instructor with a PhD in computational nano science and extensive experience as a data scientist at leading companies, you will benefit from their deep knowledge, practical insights, and passion for teaching AI and Machine Learning (ML). Join us now to embark on this transformative learning journey and position yourself at the forefront of Future-Proofing Generative AI with Vector Databases. Enroll today and unlock a world of AI innovation!
-
24Concepts of Index and Collection
-
25Index Management
-
26What is collection
-
27Index Backup Part 1: Creating Collection
-
28Index Backup Part 2: Creating Index from Collection
-
29Partitioning Vectors
-
30Upsert using Namespace
-
31Vector Partitioning Using Metadata
-
32Distance Metrics
-
33Quiz on concepts of Pinecone database management
-
34Introduction to Semantic Search
-
35Medium Posts Data Obtaining
-
36Data Preprocessing
-
37Preparing for Upsert
-
38Vector Query: "Semantic Search"
-
39Quiz on Semantic Search
This quiz tests your basic knowledge on "Semantic Search". Please review the relevant lecture if you feels like missed the basic.
-
40Concept of Named Entity Recognition (NER)
-
41NER Implementation Examples
-
42Setting up Environment for NER based Semantic Search
-
43Vector Embedding Models and Load Data
-
44Data Preparation
-
45Developing NER Helper Function
-
46Vector Embedding in Batches
-
47NER Extraction in Batches
-
48Metadata Processing
-
49Vector Upsert
-
50Vector Query: Semantic Search with NER
-
51Building an Retrieval AI Agent with LangChain and OpenAI
-
52Obtaining OpenAI API
-
53Data Load
-
54Vector Embedding Function
-
55Setup Vector DB
-
56Processing for Meta Data
-
57Embedding and OpenAI Rate Limit Workaround
-
58Indexing
-
59Semantic Search with OpenAI
-
60Embedding with OpenAI and LangChain
-
61Retrieval QA Agent- an example of retrieval augmented generation (RAG)
-
62Chat Agent